
The space target pose estimation method based on deep learning has a large amount of computation and lacks a real large dataset, and whether it can meet the high requirements of space missions for robustness has not been verified in actual space tasks. However, space applications differ from ground tasks because of their high reliability requirements and the relatively limited computing resources of space hardware. Deep learning techniques have achieved great success in many fields, especially in computer vision.
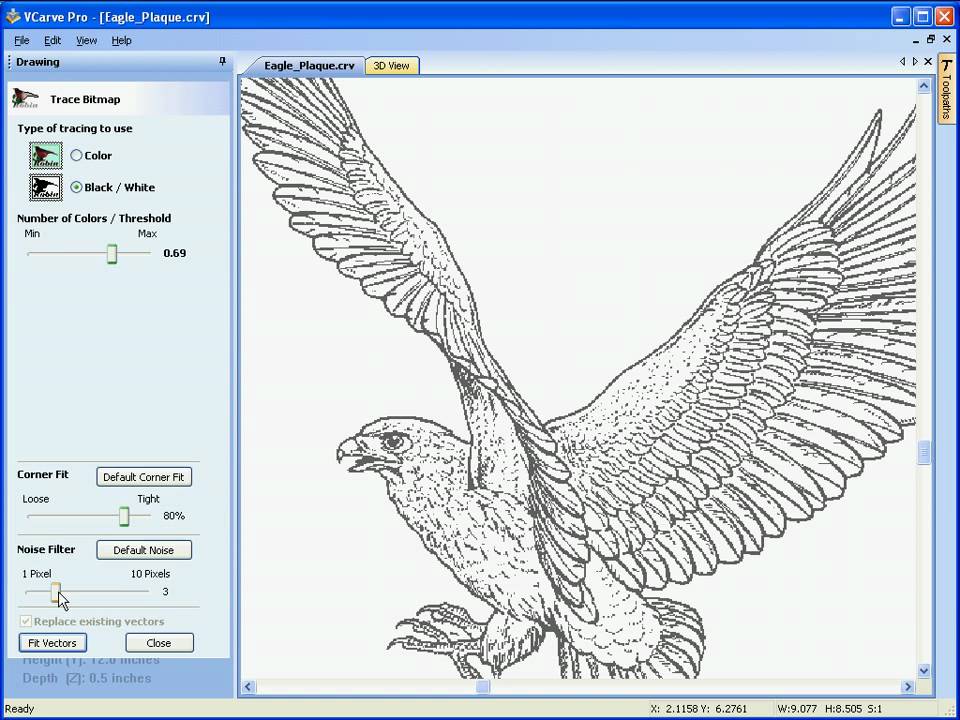
The method based on the pose estimation network is a relatively direct pose estimation method, which directly obtains the six degree of freedom pose estimation result through the overall regression and categorical voting network. The pose estimation algorithm based on the target recognition network is an indirect pose estimation method it first uses the target recognition network for feature extraction to obtain key point position information and then uses the traditional method for pose estimation. There are two main types of pose estimation methods based on deep learning: based on the target recognition network and based on the pose estimation network. Visual pose estimation methods can be divided into traditional measurement methods and deep learning methods. Vision-based measurement technology has great potential advantages in the pose estimation missions of close-range space targets. The results show that our method has better performance than traditional line segment detectors including LSD, Linelet, etc., in terms of line detection precision.Īccurate measurement of the relative pose of space targets is a prerequisite for on-orbit service missions. We demonstrate the performance of the proposed line segment detector using a variety of images collected on a semiphysical simulation platform. To further improve line segment detection’s effect, we introduce an aggregation method that uses the angle difference between segments, the distance between endpoints, and the overlap degree of segments to filter the aggregation candidate segments and connect disjoint line segments that probably came from the same segment.

An improved two-dimensional histogram construction strategy is used to optimize the Otsu method to improve the accuracy of anchor map extraction. We propose a robust line segment detector for space applications considering the complex illumination in space environments. The traditional line segment detectors show impressive performance under sufficient illumination, while it is easy to fail under complex illumination conditions where the illumination is too bright or too dark. Line segment detection is an important step in pose estimation. The relative pose estimation of the space target is indispensable for on-orbit autonomous service missions.
